By Nicole Blommendaal, in collaboration with Lea, Bijal Mehta, Marco-Dennis Moreno and Marta Ziosi.
In this article, you can find a list of interesting initiatives that work to truly make AI a tool for the social good.
Brought to you by:
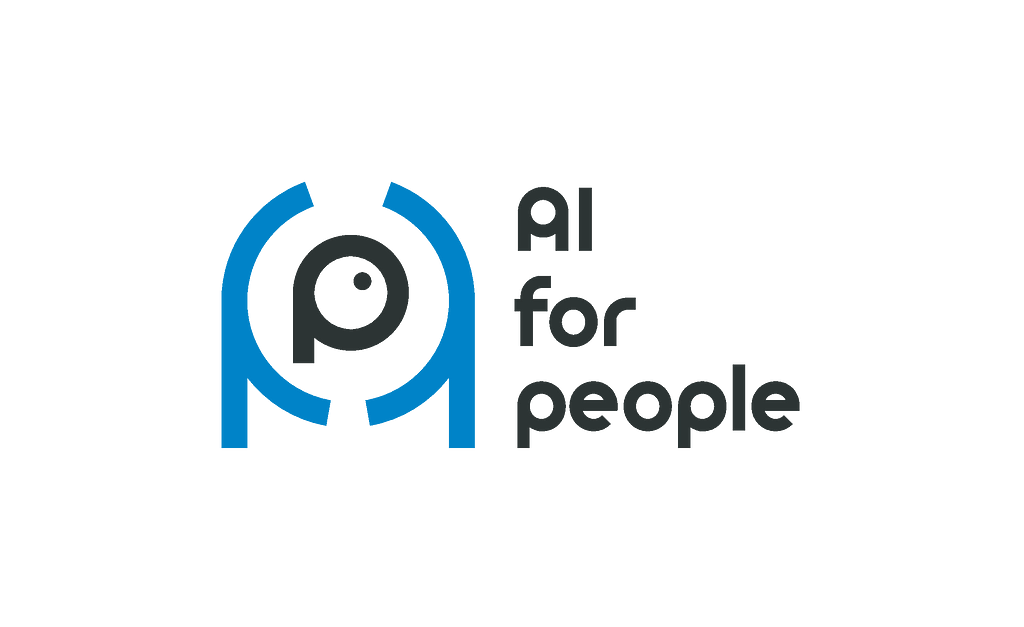
Follow us on LinkedIn, Facebook, Twitter or Instagram to check all the other projects that we launch.
There is a growing momentum coming from the academic, private, and public sectors to define what the principles by which AI should be governed and designed are. While AI systems are subject to relevant ethical concerns, the efforts of developers, governments and policy makers are alone insufficient to address those concerns in their complexity and in their consequences on the wider population.
In this respect, we think that Civil Society initiatives are key to ensuring that the most fundamental layer of society, citizens, can meaningfully shape the systems that affect them.
We, AI for People, have taken inspiration from the ethical concerns presented in the paper “The Ethics of Algorithms: Mapping the Debate” by Mittelstadt et al. (2016) to start a repository of Civil Society initiatives that are actively working on AI ethical principles. The principles are Accuracy & Robustness, Explainability & Transparency, Bias & Fairness, Privacy, and Accountability.
We here present you with a starting repository of what we consider meaningful Civil Society initiatives in the field of AI ethics, working on these principles. Often, one initiative is concerned with more than one principle, so overlap is to be expected. Most importantly, this short article is by no means an exhaustive representation of the Civil Society ecosystem.
It is rather a starting point for citizens to find out how to become active in the AI Ethics sphere and it is an invitation to other Civil Society initiatives to help us expand our repository by adding their name or other initiatives’ name here.
If you are interested, you can check-out our broader efforts on AI Ethics by visiting our website section on Ethical AI.
Without any further delay, here are the initiatives we’ve found:
On Accuracy & Robustness
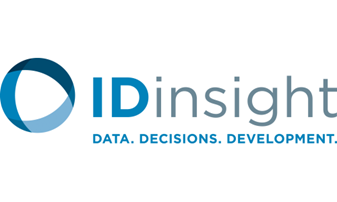
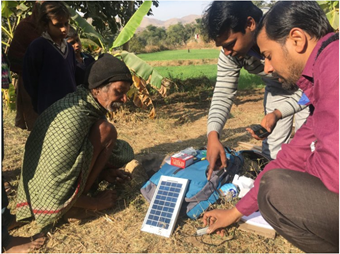
- Data on Demand is an initiative — currently focused in India with a possible future expansion to sub-Saharan Africa — by IDInsight, a research organisation identifying itself as ¨helping development leaders maximise their social impact¨, which aims to develop new approaches to survey data collection with the goal of making this collection radically faster and cheaper. Major surveys in India can take a year to implement and the wait for this data can take up to 4 years. Data on demand aims to significantly optimise this process.
- The team carries their mission out through building robust targeting tools (sampling frames) by leveraging electoral databases and satellite imagery combined with a custom machine learning model which automatically detects data quality issues and by developing a totally automated survey deployment it is aiming to provide a more efficient alternative to the current surveying system. The organisation is also building machine learning algorithms to predict in real-time which surveyors are collecting high-quality data and which need to be retrained or let go. The purpose of all of this is to increase the accuracy and efficiency of the surveying system.
- Reach them via: Email & twitter
On Transparency & Explainability

- AlgorithmWatch is a non-profit and advocacy organization based in Berlin, Germany, whose work involves keeping watch and shedding light on the ethical impact of algorithmic decision-making (ADM) systems around the world. AlgorithmWatch believes that “the more technology develops, the more complex it becomes”, but that “complexity must not mean incomprehensibility”. By explaining the effects of algorithms to the general public, creating a network of experts from different cultures and disciplines, and assisting in the development of regulation and other oversight institutions, AlgorithmWatch is driven to keep AI and algorithms accountable when they’re used in society. New and notable projects include they’re mapping of COVID-19 ADM systems and they’re 2020 Automating Society Report which analyzes ADM applications in Europe’s public sphere.
- Reach them via: Email, Twitter, Instagram and Facebook
On Bias and Fairness
- EqualAI is not only a nonprofit organization but also a movement working towards reducing unconscious bias in AI development and use. Their mission is to work together with companies, policy makers and experts to reduce bias in AI. EqualAI pushes for more diversity in tech teams and addresses existing biases in the hiring process already. They bring experts, influencers, technology providers and businesses together to write standards on how to create unbiased AI. These standards are aimed at getting brand buy-in and commitments to follow them.
- Reach them via: Email &Twitter
Other bias initiatives are: Black in AI, Data Science Nigeria, Miiafrica, Indigenous AI and Q: The genderless Voice. Other fairness initiatives are: Black Girls Code, Data Justice Lab, AI and Inclusion, Open Sources Diversity and Open Ethics.
On Privacy
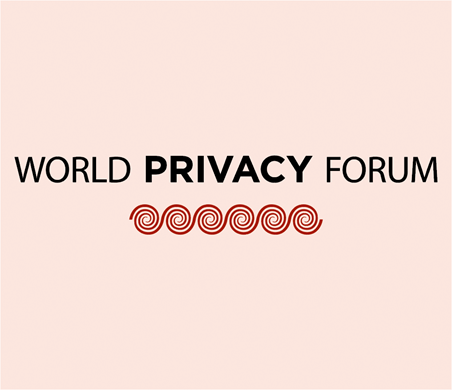
- The World Privacy Forum is a nonprofit, non-partisan public interest research group that operates both nationally (US) and internationally. The organization is focused on conducting in-depth research, analysis, and consumer education in the area of data privacy, and focuses on pressing and emerging issues. It is among one of the only privacy-focused NGOs conducting independent, original, longitudinal research. World Privacy Forum research has provided insight into important issue areas, including predictive analytics, medical identity theft, data brokers, and digital retail data flows, among others. Areas of focus for the World Privacy Forum include technology and data analytics broadly, with a focus on health care data and privacy, large data sets, machine learning, biometrics, workplace privacy issues, and the financial sector.
- Reach them via: Email, Twitter and Facebook
Other privacy initiatives are: Big Brother Watch, Future of Privacy Forum and Tor Project.
On Accountability
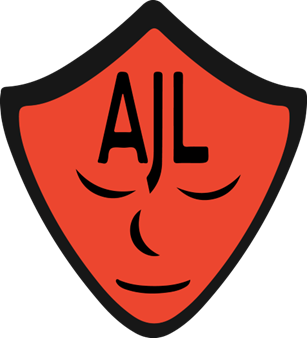
- The Algorithmic Justice League (AJL) is a cultural movement and organization that works towards an equitable and accountable AI. Their mission is to raise public awareness about the impact of AI but also to give a voice to the impacted communities. One of their core pillars is to call for meaningful transparency. Here, the Algorithmic Justice League aims to have a knowledgeable public that understands what AI can and cannot do. Furthermore, because they believe individuals should understand the processes of creating and deploying AI in a meaningful way, they too organize workshops, talks, exhibitions, and head various projects. The Algorithmic Justice League is also extremely active in the field of Bias, here below. AJL’s founder, Joy Buolamwini, is in fact part of the documentary “Coded Bias”.
- If you want to learn more about tools and resources that address a lack of transparency visit their website.
- Reach them via: Email
Other accountability-related initiatives are: Access Now, Open Rights Group, Digital Freedom Fund, and AWO Agency.
Mapping initiatives on AI ethics was originally published in AI for People on Medium, where people are continuing the conversation by highlighting and responding to this story.