New Paths for Intelligence — ISSAI19
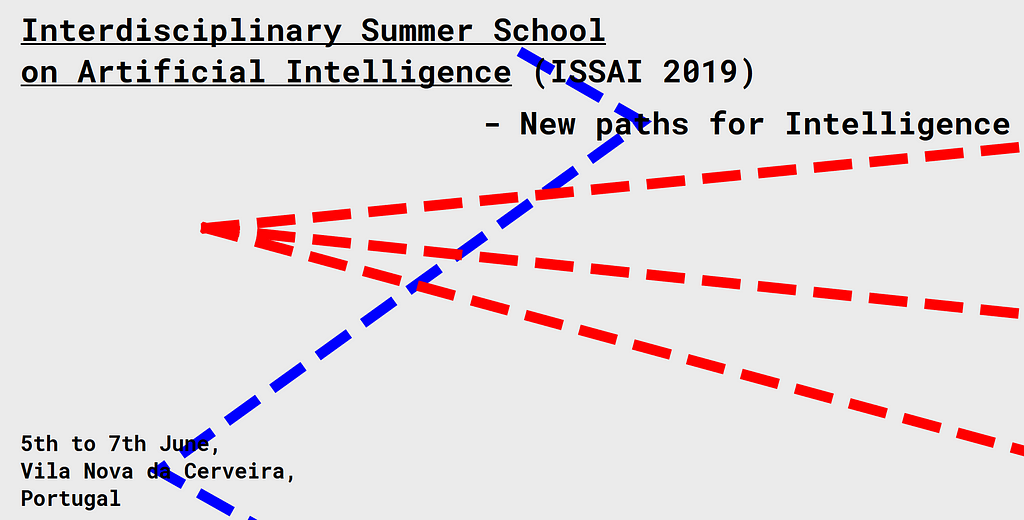
Leading researchers of the field of Artificial Intelligence met to discuss the future of (human/artificial) intelligence and its implications on society in the first Interdisciplinary Summer School on Artificial Intelligence, from the 5th to the 7th of June in Vila Nova da Cerveira, Portugal. Members of the AI for People association were present to gain a perspective on current trends in AI that reflect on societal benefits and problems. In the following article, we provide a brief overview of the topics discussed in the talks at the conference and highlight implications for societal advantages or disadvantages of AI progress. Notably, not all the talks have been summarised as we focused only on those that were considered relatable to the attending members of AI for People.
Computational Creativity
Tony Veale from the Creative Language Systems Group at UCD, provides an overview of Computational Creativity (CC). This research domain aims to create machines that create meaning. Creativity is thought of as the final frontier in artificial intelligence research [1]. Creative computer systems are already a reality in our society, whether it is generated fake-news, computer-generated art or music. But are those systems truly creative or mere generative systems? The CC domain does not aim by all means to replace artists and writers with machines, but tries to develop tools which can be used in a co-creative process. Such semi-autonomous creative systems can provide computation power to explore the creative space that would not be accessible to the creators on their own. Prof. Veale’s battery of twitter-bots aims to provoke the creation of interaction within the vibrant and dynamic twitter community [2]. The holy grail of CC — developing truly creative systems, capable of criticising, explaining and creating their own masterpieces — is still considered at debatable reach.
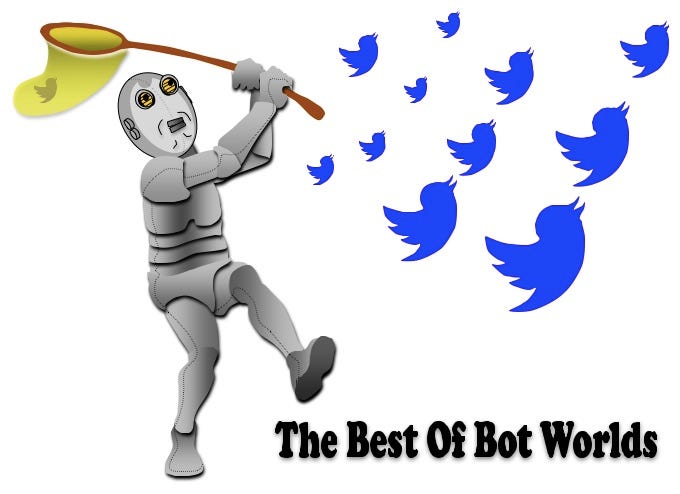
Implications: We see Artificial Intelligence as something logical, reasonable and efficient. Often, we associate its influence with economy and technology. We might overlook that the domain of creativity, which is in its core a developing society within its culture, art and communication, equally affected by AI. We need to become aware of this influence, which works on the one hand in favour of the creative human potential by providing powerful tools that can help us develop new ideas. On the other hand, there is the potential of underestimating this creative influence and falling for fake-news and alike. The former is the benevolent use of CC, whereas the latter is the malicious (ab)use of CC.
Machine Learning in History of Science
Jochen Büttner from the MPIWG Berlin presented new tools for a long-established discipline: Using machine learning approaches for corpus research in the history of science. Büttner presents the starting point as the extraction of knowledge from the analysis of an ancient literature corpus. Conventional methods, e.g. manual identification of similar illustrations among different documents, are highly time-consuming and seen as impractical. However, machine learning techniques provide a solution to such tasks.
Büttner explained how different techniques are being utilised to detect illustrations on digitised books and identify clusters of illustration, based on the use of the same woodblocks in the printing process (shared between printers or passed on).
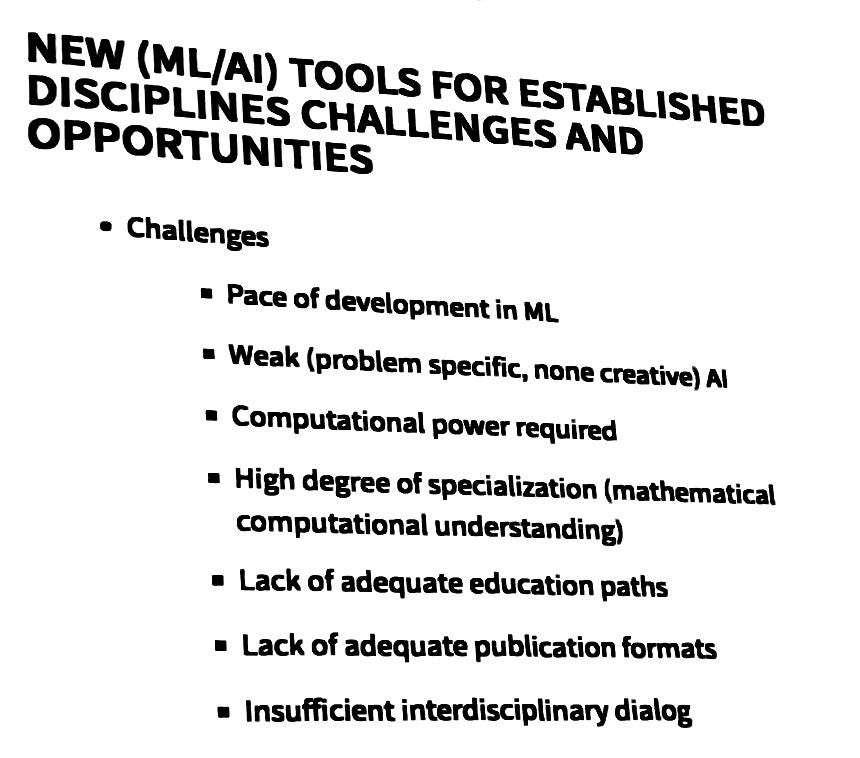
Implications: The research provides an interesting example of how one research field (history of science) can greatly benefit from another (artificial intelligence). With only 6 months of AI experience Prof. Büttner can achieve results that otherwise would be years of effort. Yet, from an AI perspective, the implementation is rather naive. The resulting divergence of abstract machine learning research with actual applications in other domains is clear as specialised algorithms could be used to yield better results. Challenges addressed by the talk are the rapid pace of development in ML, which already seems to be overwhelming when specialising only on Machine Learning. Overall, ML requires a rather high demand in mathematical computational understanding, which makes it even harder for foreign domains to gain access. Therefore, it is key to provide adequate educational paths for everyone and encourage the application of AI by establishing adequate publication formats, which will in return foster interdisciplinary dialogue.
Artificial Intelligence Engineering: A Critical View
The industry talk had been given by Paulo Gomes Head of AI at Critical Software. Gomes provides insights from someone who has worked for years in research switching to industry. The company is involved in several projects that use Machine Learning: identification of anomalous behaviour in vessels (navigation problems, drug traffic, illegal fishing), prediction of phone signal usage to prevent mobile network shutdowns, optimization of car manufacturing energy consumption or even decision making in stress situations in the military context. The variety of addressed domains shows the range of involvement of AI in our ‘technologised’ society.
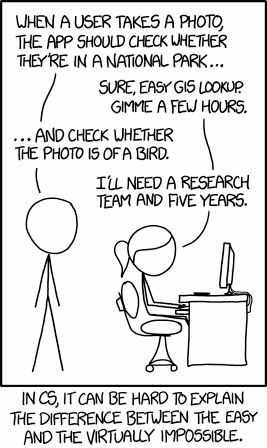
Implications: The talk also addresses the critical gap between what companies promise and what is actually possible with AI. This gap is not only bad to the economy, but directly harmful to people. As AI will grow, the expectations have already grown much higher than what can be achieved in neither research nor industry. The AI-hype about the massive leaps in technology due to recent developments in deep learning are somewhat justified, triggering a “New Arms Race for AI” [3] between the USA, Russia and China. The talk points out that this technological bump fits into the scheme of the hype cycle for emerging technologies with Deep Learning as the technology trigger (see image).
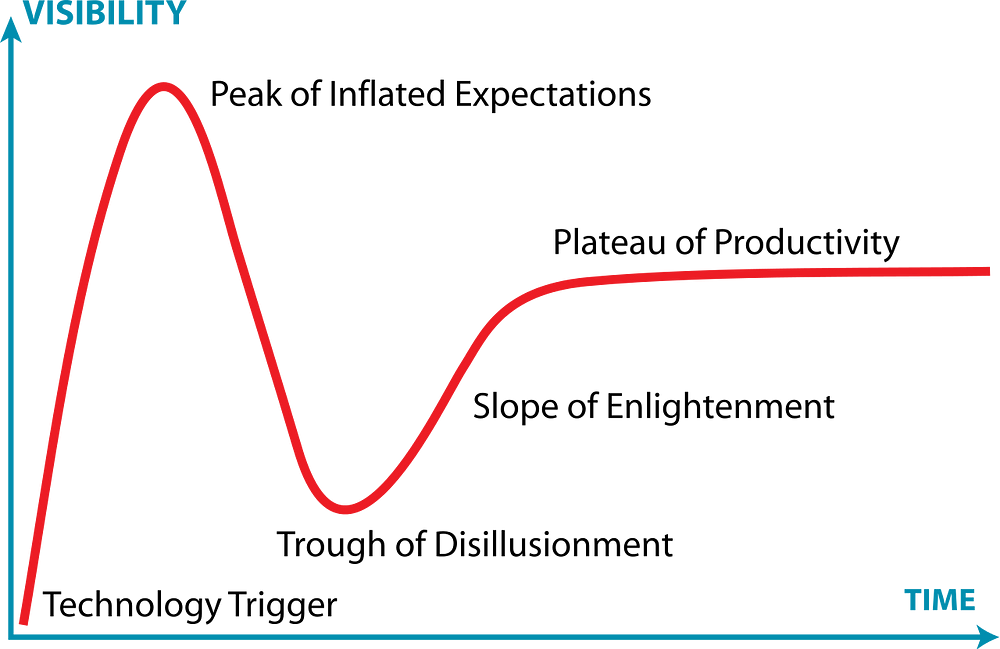
Suddenly, every company needs to open up an AI department even though there are too few people with the actual experience in the field. A wave of job quitting and career swapping is currently being observed. Nonetheless, in most cases people find themselves with little field experience in a company that has even less — low knowledge growth and lack of appreciation due to little understanding from the company’s side. These people might end up jumping in front of rather than on top of the AI hype train.
Why superintelligent AI will never exist
This talk was given by Luc Steels from VUB Artificial Intelligence Lab (now at the evolutionary biology department at Pompeu Fabra University). In a similar fashion to the previous talk, Steels outlines the rise of AI technologies in research, economy and politics. The cycle is described in a somewhat different way and can be found in various other phenomena: Climate change has been discussed for decades, but it had been given very little actual attention in politics and economics. It is only when people are faced with the immediate consequences that politics and economics start to pick it up. In the race for AI technology, we can observe this first underestimation by a lack of development, i.e. for a long time AI struggled with its establishment in the academic world and found little attention in economy. Now, we are facing an overestimation in which everyone is creating higher and higher expectations. Why is it, that the promised superintelligent AI will not exist? Here are a few examples and implications from Steels:
- Most Deep Learning systems are very dataset-specific and task-specific. For example, systems that are trained to recognize dogs, fail to recognize other animals or dog images that are turned upside-down. The features learned by the algorithm are irrelevant when it comes to human categorization of reality.
- It is said that these problems can be overcome by more data. But many of those problems are due to the distribution and the probability within the data and those will not change. That is, those systems do not learn global context, even if presented with more data.
- Language systems can be trained without a task and can be provided massive amounts of context. Yet, language is a dynamic, evolving system that changes strongly over time and context. Therefore, language models would lose their validity quickly unless they are retrained on a regular basis, which is a ridiculously effortful computation.
“A deep-learning system doesn’t have any explanatory power, the more powerful the deep-learning system becomes, the more opaque it can become. As more features are extracted, the diagnosis becomes increasingly accurate. Why these features were extracted out of millions of other features, however, remains an unanswerable question.”
Geoffrey Hinton, computer scientist at the University of Toronto — founding father of neural networks
- The systems learn from our data, not our knowledge. Therefore, in some cases these systems do not apply any sort of common sense and take our biases into their models. For example, Microsoft’s Tay chatbot that starting spreading anti-semitism after only a few hours online [4].
- Reinforcement learning algorithms are implemented to optimize the traffic on a web-page and not to provide content. Consequently, click-baits are more valuable to the algorithm than useful information.
Conclusion
This summer school was the first of its kind, a collaboration of the AI associations from Spain and Portugal. Despite the reduced number of participants and the lack of female speakers, this first interdisciplinary platform for the AI community provided a basic discussion about the implications of AI and its future. More people should be educated about the illusionary expectations created by the AI hype in order to prevent any damage to research and society. The author would like to thank João M. Cunha and Matteo Fabbri for their contributions to this article.
References:
[1] Colton, Simon, and Geraint A. Wiggins. “Computational creativity: The final frontier?.” Ecai. Vol. 12. 2012.
[2] Veale, Tony, and Mike Cook. Twitterbots: Making Machines that Make Meaning. MIT Press, 2018.
[3] Barnes, Julian E., and Josh Chin. “The New Arms Race in AI.” The Wall Street Journal 2 (2018).
[4] Wolf, Marty J., K. Miller, and Frances S. Grodzinsky. “Why we should have seen that coming: comments on Microsoft’s tay experiment, and wider implications.” ACM SIGCAS Computers and Society 47.3 (2017): 54–64.
New Paths for Intelligence — ISSAI19 was originally published in AI for People on Medium, where people are continuing the conversation by highlighting and responding to this story.